So, you are in charge of the marketing budget and need to evaluate which programs work better so you can do more of it next year. Sounds easy, right? You did some A/B testing, looked at your control vs. your treated groups and figured it out. Then, why is it not really working? Your comparison was not apples-to-apples and you failed to properly account for differences between the two groups. Let’s explain the issue below and go over strategies to fix it and understand what truly works.
A simpler case first: helping smokers live longer
Let’s start with a case drawn from the medical industry for its simplicity and ease of understanding. You are tasked with evaluating whether a lung cancer drug works. The mortality rate of the control group is about 24% while your treated group shows 35%. You might conclude the drug does not work, which would be incorrect.
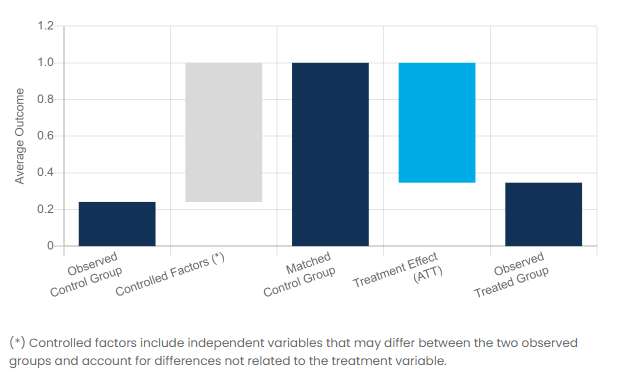
In reality, the control and treatment groups are not comparable: the people treated with the drug included a lot more smokers (52% vs. 21%) and were much more at risk, which is why they were treated in the first place. The control group was essentially healthier.
Once you account for differences in smoker populations between the two groups, you can create an adjusted control group that matches the demographics of the treated group. You then find out the drug is actually very effective, which is the opposite of the conclusion a naïve analyst would have drawn!
Analyzing marketing effectiveness programs
Let’s now turn our attention to a marketing use case from the Telecom industry and let’s see how we can use A/B testing analysis to better understand what marketing programs are effective at retaining customers. In this case we are trying to decide whether promoting online backup services is an effective strategy to increase overall customer retention.
The hypothesis is that the more products we sell, the more likely we are to retain a customer. The marketing team decides to compare churn rates (the rate at which customers cancel service) between two groups: a group of customers who purchased online backup services, and one with customers who didn’t.
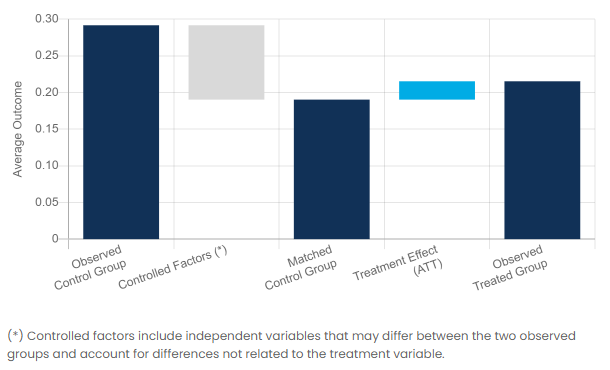
Here again, appearances can be deceiving: the treated group (who purchased backup services) shows a churn rate that is about 25% lower than the control group. The gotcha here is that the two groups are not really comparable: the treated group over-indexes significantly on older, married customers on annual contracts. Once you adjust for these differences you find out that, all else being equal, purchasing a backup service leads to more customers leaving, not fewer.
How does A/B testing analysis work?
Proper A/B testing analysis relies on body of modern techniques known as causal analysis. At its heart, A/B testing boils down to reconstructing after the fact a proper control group with attributes (demographic and other) that match closely the attributes of the treated group. Doing so with a rigorous approach allows us to remove biases associated with factors that have nothing to do with the marketing program we are trying to evaluate.
There are many different techniques for this, including propensity score matching, propensity score weighting, propensity score blocking, propensity score stratification, and ordinary least squares. It is typically implemented with a team of data scientists using tools such as R or Python libraries. In a related post we cover how business analysts can do A/B testing analysis in minutes using the G2M platform.
How Can We Help?
Feel free to check us out and start your free trial at https://app.g2m.ai or contact us below!