Pricing is one of the biggest levers you can pull to affect business results. It is typically set by businesses using some version of the following planning cycle:
- Pricing strategy: the team sets a pricing strategy in support of business objectives, e.g. be the low-cost provider to drive market share
- Demand analysis: collect any and all sources of pricing information (typically internal or trade data), conduct ad hoc analysis
- Competitive analysis: collect external sources of competitive information to understand market and segment pricingdynamics, conduct ad hoc analysis
- Profitability analysis: analyze customer and product profitability for different price points
- Offer development: design a product- and customer-specific offer
- Trialing and reporting: field different pricing trials in market to measure actual demand for specific offers and price points
- Feed results back into strategy above
Now the problem with this historically is the pricing planning cycle is long, imprecise, labor intensive, and very much ad hoc. In many industries good data was, until recently, missing, and the means to analyze demand in real time were simply not available. The growth in data, cloud computing, and AI technologies is completely changing this paradigm.
Historically pricing analysis was based on limited data,
and conducted in a slow, delayed manner. AI now enables us to
price at scale, in real-time, and in a very granular manner,
with meaningful profit impact.
The first major catalyst is the availability of large-scale, granular demand data. We can now collect and build demand curves encompassing dozens if not hundreds of millions of prospects with known willingness to pay. The second enabler is the availability of AI technology to generate and analyze detailed demand curves at scale, providing optimal pricing decisions in real-time at a customer level.
These advances truly enable modern marketers to move from a traditional view of demand that is high-level, mostly static, to a highly surgical view at the customer or prospect level that enables unprecedented pricing accuracy. Let’s explore two examples to understand the benefits of technology applied to pricing.
In the first example a B2B services provider was looking to significantly increase market share to drive top-line growth with a fixed cost model. Using a combination of external and internal data sources they built a large database of prospects including spending information and serviceability, enabling the development of extremely detailed demand curves one prospect at a time. This in turn enabled the operator to analyze and predict product mix shifts and growth across the product line and markets, leading to a 50+ percent increase in sales.
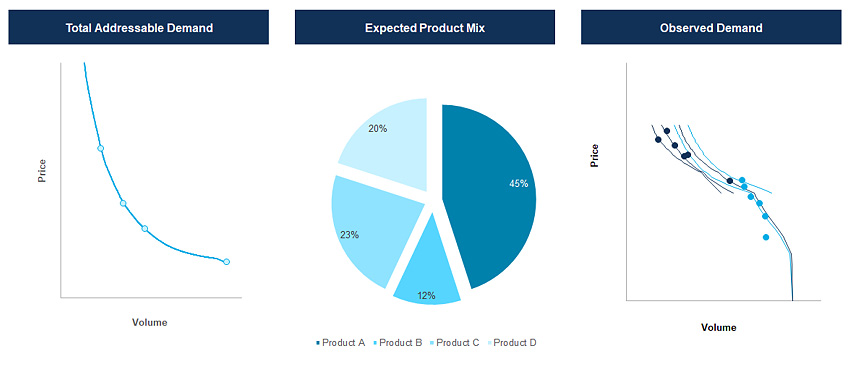
In our second example an online recruiting firm was faced with highly heterogeneous demand for its services, driven by a broad spread of attributes within its prospect base such as income, and willingness to pay. Instead of using its legacy uniform pricing strategy the firm developed a targeted pricing policy using AI technology, specifically a combination of LASSO with a weighted likelihood bootstrap (WLB) to provide optimal, real-time targeted pricing decisions at a customer level. This approach improved revenue by over 80% relative to the legacy approach, with most customers being charged less than the original, uniform price.
How Can We Help?
Feel free to check us out and start your free trial at https://app.g2m.ai or contact us below!